Authors
D.M. Vidmar, W. Thompson, K. Morland, G. Lee, R. Miotto, J. Leader, J. Pfeifer, B. Fornwalt, A. Nelsen, R. Chen
RATIONALE: Accurately identifying patients with pulmonary hypertension (PH) through high-quality phenotyping is critical for defining clinical research cohorts and generating accurate labels for machine learning (ML) models. However, PH is often poorly captured in observational datasets due to known limitations of structured data such as diagnosis codes or problem lists. Therefore, we developed a novel phenotyping pipeline using both structured and unstructured electronic health record (EHR) data for the development and validation of an improved PH phenotype.
METHODS: Using a longitudinal de-identified EHR dataset of over 2 million patients, we first created and iteratively improved a structured phenotype using various data elements including diagnosis codes, problem lists, procedures, medications, and imaging results. We then developed labeling functions-a collection of logic rules and regular expressions to determine whether patients are likely to have PH-to generate weak labels. These labeling functions integrated multimodal signals from structured data, unstructured note data, and diagnostic study reports. Then, we used the weak labels to train a state-of-the-art transformer-based natural language processing (NLP) model. All structured and ML phenotypes
were iteratively developed on a training data set and then evaluated on an independent test set; both training and test sets underwent blinded, manual chart review by clinicians (total N=600).
RESULTS: We identified 39,602 unique patients with PH based on our final and best-performing phenotype, which used a combination of NLP model output and structured data. This phenotype achieved a 91% positive predictive value (PPV), 76% sensitivity, and 0.83 F1 score on our independent test set. For comparison, our best-performing structured phenotype had a similar PPV (93%), but only a sensitivity of 54% (Table). NLP models using only note text significantly outperformed phenotypes using structured data elements from the EHR at all iterative stages of development. Still, the combination of the two ultimately generated the best performance. The overall pipeline was easily scalable and efficient, resulting in total iterative development times comparable to traditional structured phenotype development alone.
CONCLUSIONS: Using a novel phenotyping pipeline, we developed a high-quality, accurate phenotype for identifying patients with PH in EHR data, leveraging both structured EHR data and the richness of unstructured note data, as validated through blinded chart review. By better identifying the disease populations of interest, such performant phenotypes will facilitate higher-quality observational research studies and allow for better training and evaluation of ML models, thereby improving performance and generalizability.
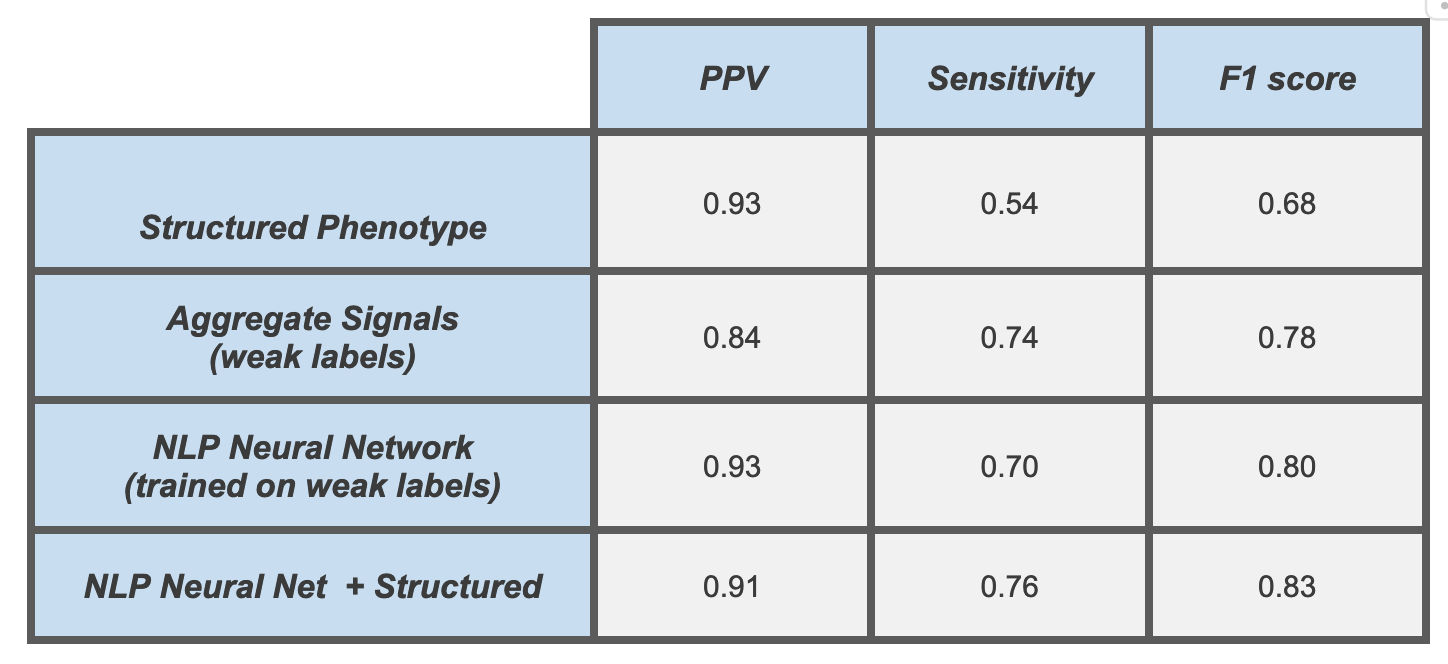
VIEW THE PUBLICATION
VIEW THE POSTER