Authors
G. Lee, N. Hakim, R. Chen, J. Pfeifer, D. Vidmar, W. Thompson, A. Nemani, R. Miotto, K. Morland, A. Nelsen, B. Fornwalt
RATIONALE – Patients with pulmonary hypertension (PH) often suffer diagnostic delays and many remain undiagnosed. Machine learning (ML) models using electrocardiograms (ECGs) may reduce these diagnostic gaps but face two major challenges: 1) lack of high-quality labels due to poor-performing phenotypes-limiting model training and downstream evaluation; 2) models trained only on patients with both ECGs and echocardiograms in close time proximity may be overfit and fail to generalize to broader, intended use populations. We trained an ECG-based ML model to identify patients at high risk for PH using an improved PH phenotype and validated the model on a true intended use population – all patients with ECGs without a pre-existing PH diagnosis.
METHODS – Using a dataset of 3.1M ECGs from 648K patients, we linked 2.8M ECGs with 628K patients; 34,346 patients with 461K ECGs were positive for PH based on a previously validated NLP-based phenotype. Data were split into a train, development, operating point set, and an independent test set for final model evaluation. We trained models to predict whether patients without pre-existing disease would develop PH. We tested multiple prediction windows (6, 12, and 18 months), model architectures, and operating points. We evaluated the model on the independent test set using the area under the receiver operating curve (AUROC), the area under the precision-recall curve (AUPRC), F1 score, and measured sensitivity, specificity, positive predictive value (PPV), and negative predictive value (NPV) for individual operating points. We also evaluated performance across subgroups including age, sex, and race/ethnicity.
RESULTS – The final model, using a convolutional neural network architecture, performed well in identifying patients at high risk for PH within 12 months, with AUROC of 0.86, AUPRC of 0.25, and F1 score of 0.31. We chose an F2-optimized, sensitivity-favoring operating point with 92% specificity, 54% sensitivity, 22% PPV, and 98% NPV (Figure). At this point, the model predicted 10.4% of patients as high risk, with >1 in 5 being true positives. For comparison, an F1-optimized operating point had lower sensitivity (32%) but higher PPV (30%), predicting 4.6% of patients as high risk. Performance was similar across all subgroups and time windows. For a medium-sized health system of 1M patients, these models may conservatively identify 112-421 new cases of PH annually.
CONCLUSIONS – An ECG-based ML model trained using validated NLP-based phenotype labels can identify patients at high risk of PH in a true intended-use population.
These findings warrant further prospective validation.
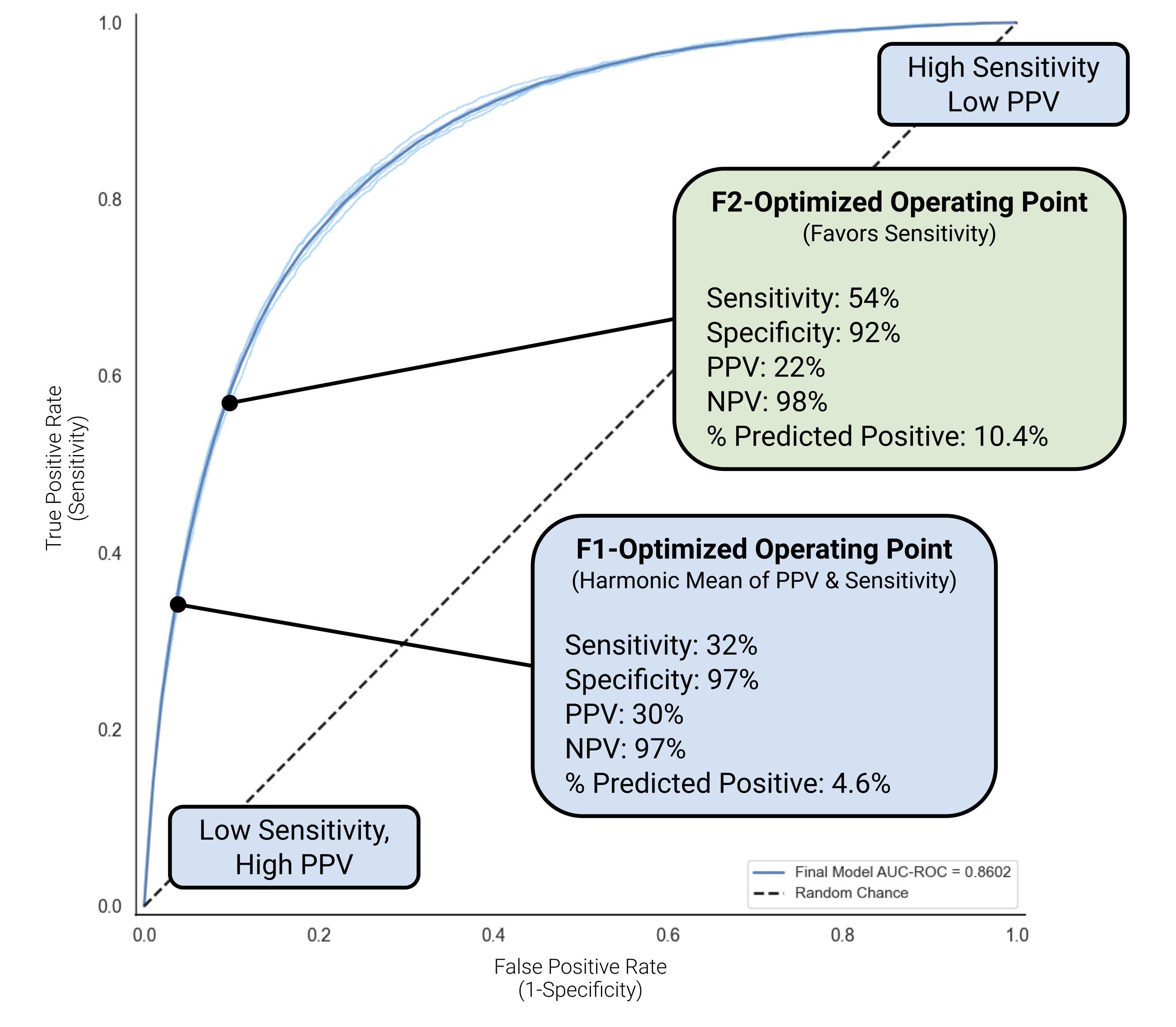
VIEW THE PUBLICATION
VIEW THE POSTER