Authors
R. Chen, J. Pfeifer, D. Leibowitz, D.M. Vidmar, W. Thompson, J. Leader, K. Morland, A. Nelsen, B. Fornwalt
Rationale – Pulmonary hypertension (PH) is a progressive, debilitating disease with increasing treatment options. However, identifying PH patients in real-world datasets is challenging, thereby limiting efforts to optimize medical therapy and improve clinical research, including generating accurate estimates of population-wide prevalence. We sought to determine whether patients with PH could be reliably identified using structured electronic health record (EHR) data and estimate population-wide prevalence.
Methods – Using a longitudinal de-identified EHR dataset of over 2 million patients, we first developed a filtering method to remove patients without any evidence of disease or possible disease across multiple data modalities. This enables random sampling for a reliable estimate of sensitivity within a feasible number of chart reviews. From the remaining cohort(filtered population), we randomly sampled 300 patients for a blinded, two-physician review to identify those with clinically diagnosed defined as a clear diagnosis or mentioned in the unstructured note text by a clinician. We then characterized the availability of structured data elements, including any occurrence of a PH diagnosis code on the problem list or in any encounter, any order or medication reconciliation of PH-specific vasodilator therapies, or any right heart catheterization (RHC) with mean pulmonary artery pressure >20mmHg. Additionally, we calculated an estimate for population-wide prevalence by extrapolating the proportion of true positives within the random sample of the filtered population to the entire EHR population; this conservatively assumes all patients outside the filtered population are disease-free, ensuring we do not overestimate true prevalence.
Results – Within the random sample of the filtered population, we identified 108 patients with clinically diagnosed PH, of which only 57%(62/108) had any structured data elements suggestive of PH (Figure). Specifically, only 26% of patients had a PH code on their problem list, 53% had a PH encounter diagnosis code, 2% were on a PH medication, and 12% had a RHC meeting diagnostic criteria. Overall, 43% of patients did not have any structured EHR data suggestive of PH yet were noted to have clinically diagnosed PH in unstructured note text. The population-wide prevalence of PH was estimated to be 2.4%, which is higher than the∼1% estimate in existing literature.
Conclusions – Structured data elements in EHR datasets fail to identify a large proportion of patients with clinically diagnosed PH. Leveraging unstructured data from clinical notes, such as in natural language processing-based phenotyping approaches, may be necessary for more complete identification of patients with PH in EHR data.
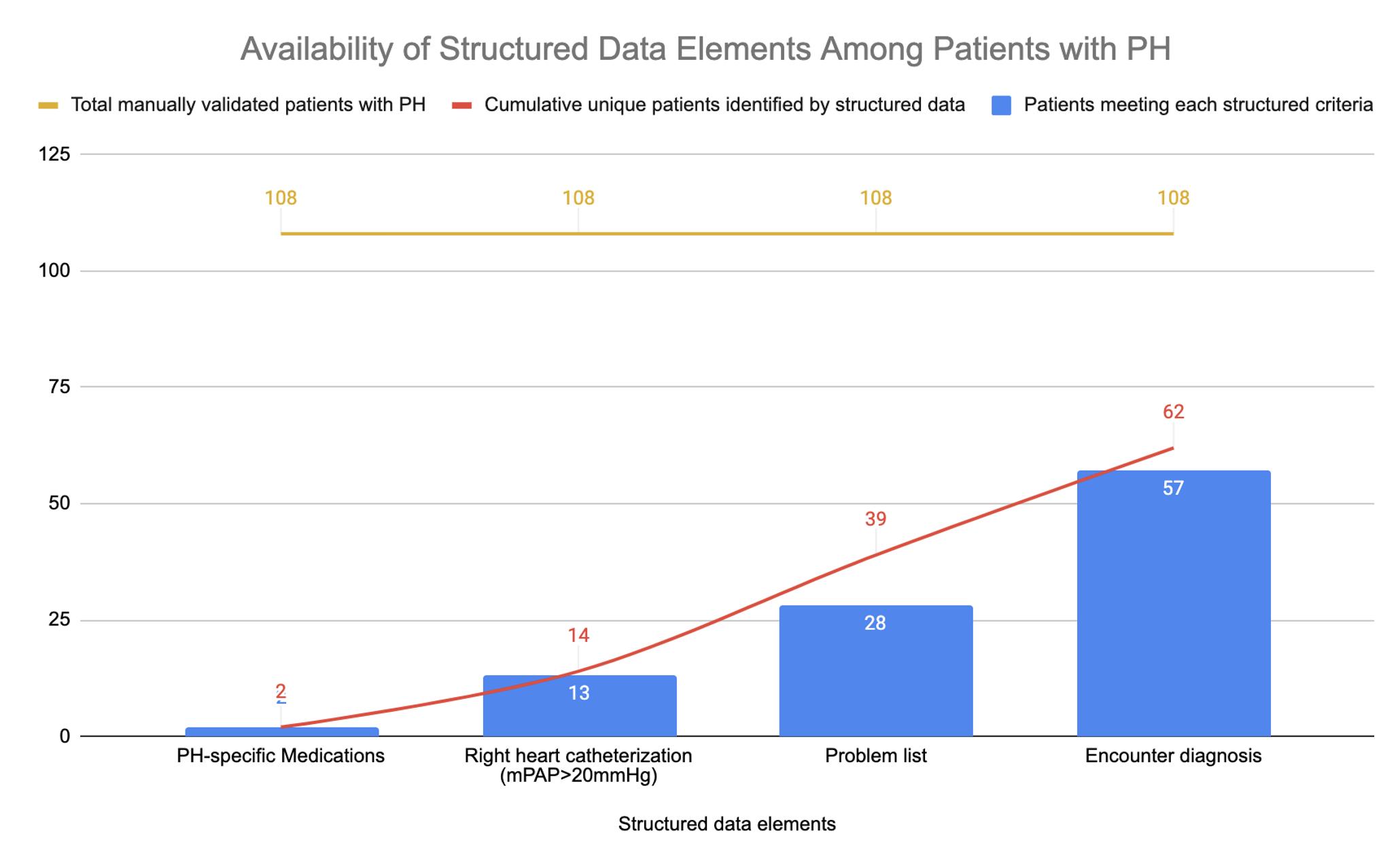
VIEW THE PUBLICATION
VIEW THE POSTER